AI speeds up the diagnosis of urinary tract infections
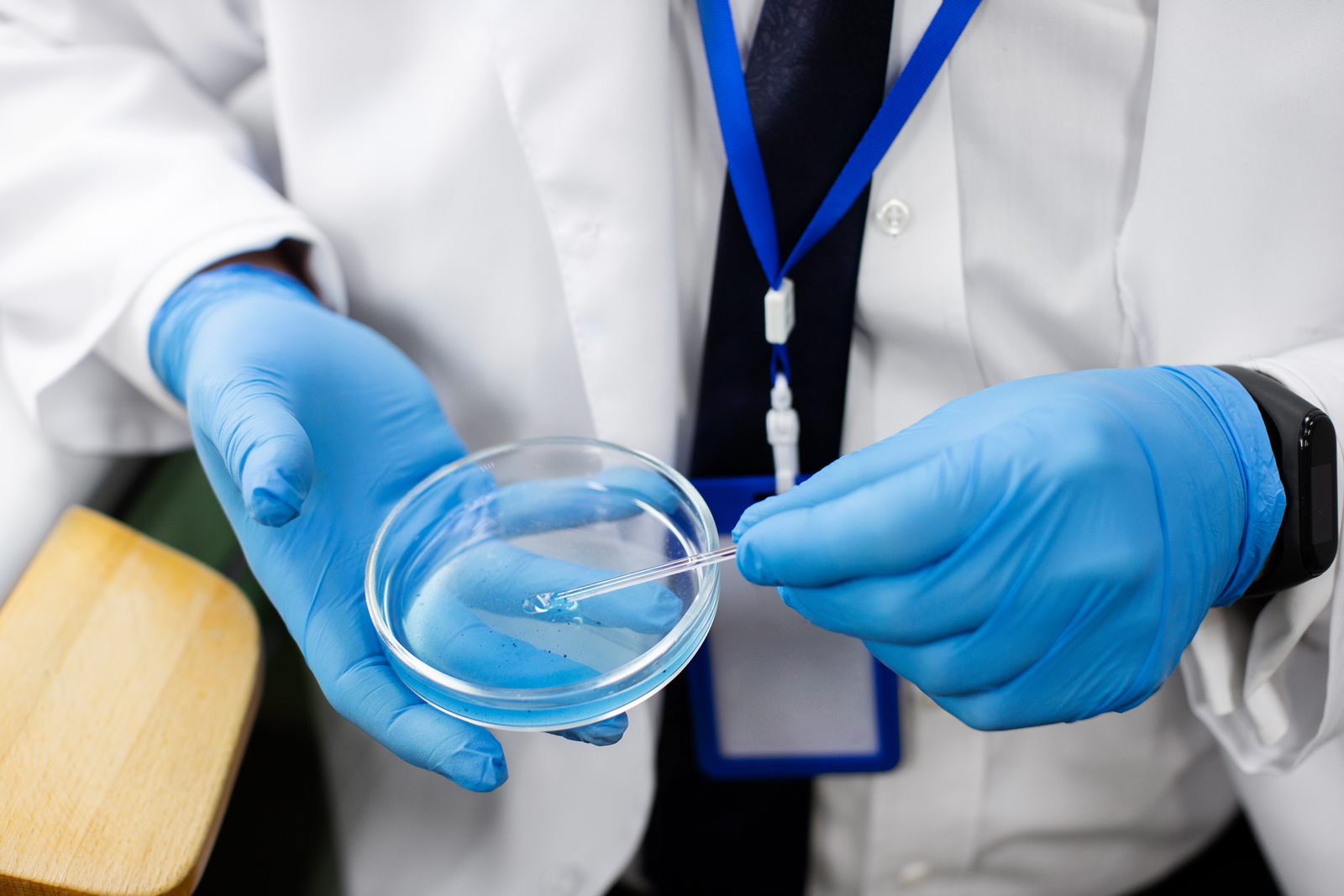
Fraunhofer Austria and the AULSS2 Marca Trevigiana Institute in Treviso have developed a method based on artificial intelligence that can reduce the workload of laboratories
Urinary tract infections are usually diagnosed by means of urine culture: an extract of the urine sample is applied to a plate and examined for bacterial growth under a microscope for the following 24 to 48 hours. After this time-consuming procedure, however, more than two thirds of samples turn out to be negative. Being able to filter out these negative samples in advance would significantly reduce the workload in laboratories, and negative test results would be available much more quickly. The artificial intelligence (AI) now developed by the Austrian and Italian research team can detect negative samples more accurately than previous methods and can therefore reduce the workload in the laboratory by 16 percent. The algorithm used is falls in the category of the so-called interpretable AI: it informs the doctors treating the patient of the reasons why it identified a sample as negative. The results of the study were published in the "American Journal of Clinical Pathology" and are available for immediate use on suitable machines.
As it is very time-consuming to examine every urine sample in detail, many hospitals already use flow cytometry. In this way, doctors are able to make a pre-selection so that obviously negative or contaminated samples are not sent to the urine culture in the first place. A widely used device for this purpose is the "Sysmex Uf-1000i", whose data the researchers analyzed as part of their study. This device counts and classifies particles in the sample automatically and outputs more than 40 parameters that can then be used for diagnosis. Previous methods for identifying negative samples, however, used only a few of these parameters - a fact that provided the impetus for the research project. "We wanted to see whether we can improve the results by including more parameters in the assessment instead of only two or three," explains Giacomo Da Col, head of the project at Fraunhofer Austria. Together with medical doctor Fabio Del Ben from the National Cancer Institute in Aviano, Italy, and a research team, 15,312 samples from 10,534 patients were evaluated.
Decision trees as interpretable AI
However, the researchers had special requirements for the AI to be used in the project: "Especially in medicine, it is important that an AI is not a black box. It makes no sense for an AI to just give an assessment without providing an explanation. Therefore, it was a mandatory requirement for us that our algorithm is transparent and interpretable and that doctors know why a sample was categorized as negative," explains Giacomo Da Col. One form of AI to which this applies are the so-called decision trees. This methodology is very intuitive and makes it possible to understand its assessments.
The way decision trees work is comparable to the way humans think: it asks and answers certain questions one after the other in order to reach a judgment. The team found that the AI's decision criteria were very similar to those of doctors. The AI also concluded that one of the most important criteria had to be the number of bacteria, closely followed by the patient's age. Unlike previous methods, however, the researchers also took other parameters into account. The final algorithm uses seven of the available parameters, has the required sensitivity of 95% and can reduce the workload of laboratories by 16% compared to previous methods.
Several rounds of refinement were necessary to achieve this result. "We made several improvements to the decision tree algorithm to increase performance while maintaining interpretability," explains Doriana Cobârzan from Fraunhofer Austria, who played a key role in the development of the AI.
Since the entire decision tree was published in the article in the American Journal of Clinical Pathology, users who use the same flow cytometry device as the research team can immediately program the methodology into their machine and apply it in medical practice right away.
Cooperation partners wanted
A drawback of the study, according to project leader Giacomo Da Col, is that it has so far only been carried out at one single hospital. The researchers are therefore looking for cooperation partners who would like to carry out a similar study at their hospital. "If, for example, the sampling procedure is different in another hospital, the results may differ. People's diet also has an influence on the analysis of urine samples, so in a region where people have a different diet the results may differ slightly. It would be desirable to evaluate the algorithm in other hospitals," explains Giacomo Da Col.